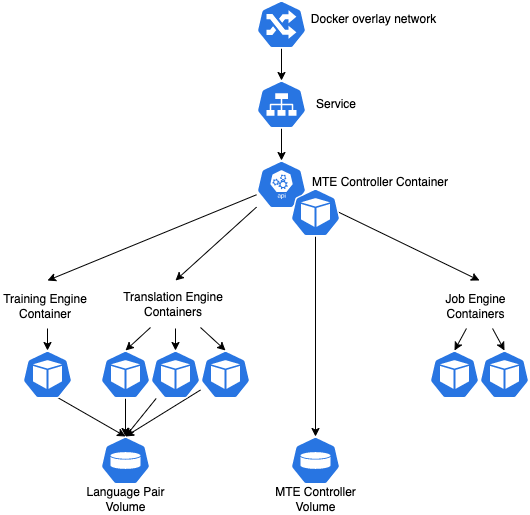
- Docker host with CPU/RAM totalling up to the minimum requirement.
e.g. Azure, EC2, VMWare - Docker & Docker-Compose installed on the host.
- Sudo access granted to user mtedge.
- Recommended size of the local storage for the image & Language Pair models.
- Edge Controller host is deployed as a container in docker and Edge UI & API are published as a service in the docker overlay network.
- Job Engines, Translation Engines & Training Engines are deployed as containers and could be manually configured to scale up/down based on the predefine number of containers defined in the docker-compose.yml file.
- Language Pairs and the Edge configuration is saved in docker volumes.
- All containers use the same docker base image.
- Prerequisites
- Create mtedge user & group with the id 10001.
$ groupadd mtedge -g 10001
$ useradd mtedge -u 10001 -g 10001 -m -s /sbin/nologin
- Grant sudo access to user mtedge.
eg. on a Ubuntu EC2 host, edit /etc/sudoers.d/90-cloud-init-users file and add new line as below
$ vi /etc/sudoers.d/90-cloud-init-users
mtedge ALL=(ALL) NOPASSWD:ALL
- Install docker & docker-compose on the host.
- Create mtedge user & group with the id 10001.
- Helm charts & example “values.yaml” files are provided by RWS for easy deployment.
- Upgrades & rescaling of Edge configuration is possible with helm upgrades.
- Training Engines require GPU nodes in the Kubernetes cluster for better performance.
- LW Edge license & default admin accounts are saved as Kubernetes secrets.
- Persistent volume of the Controller pod could be a standard RWO storage class.
- Persistent volume of Language Pairs requires a high performance, case sensitive RWX storage class.
- Language Pairs are deployed to the persistent volume using Kubernetes Jobs.
- Bootstrap Language Weaver Edge admin user.
- Install new Language Pairs.
- Increase/Decrease the size of the pool of Job Engines, Training Engines or add/modify the pool of Translation Engines for a given Language Pair.
- Auto scale the number of Translation Engines, depending on the system load.
- Controller pod.
- Job Engine pods - all pods share the same environment variables.
- Translation Engine pods - each Language Pair has its own environment variables.
- Training Engine pods - all pods share the same environment variables.